09.01.23ai aichatbot openai chatgpt
PART 2: IMPLEMENTING AI IN YOUR ORGANIZATION
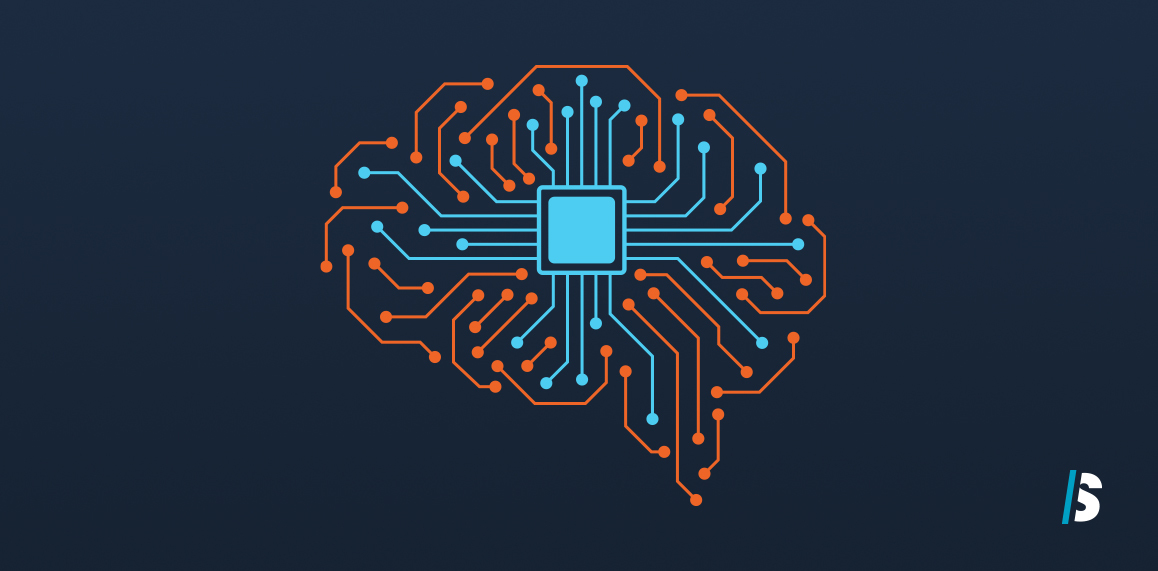
The interest in AI technology has never been as heightened as it is now. While the concept of AI technology has been around for over 60 years, companies like OpenAI have revitalized AI technology by introducing ChatGPT. This has led many companies to look for opportunities to use AI technology to help their business. However, having an interest in utilizing AI technology and actually implementing it is sometimes more complicated.
There are many things to consider when approaching an opportunity for AI technology to provide a solution. The first step is understanding that there are three types of AI capabilities and identifying the capability that more closely aligns with your solution.
AI Capabilities
Chatbots, drafting communications, optimizing delivery routes for drivers, personalized customer service, and recommendation engines are all examples of solutions where AI technology can have an impact. All of these solutions fall into three types of capabilities: data analysis, customer and employee engagement, and process automation. These capabilities have been identified based on value and effort.
Data Analysis
Analyzing data is a task that can be completed by humans quite well and is often done by employees at companies. However, doing so can take quite a long time, especially if a ton of data needs to be reviewed. AI technology is equipped to handle this and save a company time. However, producing an adequate AI solution in data analysis takes much effort. Solutions that fall into this capability are usually high-value / high-effort solutions. Examples are predicting what a customer is likely to buy, detecting credit card fraud, gauging customer reactions, and evaluating safety.
Customer and Employee Engagement
Customer engagement can be the difference between a good company and a great one. Providing 24/7 customer service, personalizing the customer’s experience, and recommending options and solutions are some of the most common ways of utilizing AI technology.
On top of creating AI solutions for customers, the ability to better support employees also exists. For example, solutions that help onboard new employees, help train less experienced employees, or coach employees as they work are just some of the opportunities that companies have been implementing with AI technology.
While these customer and employee engagement opportunities exist for utilizing AI technology as a capability, opportunities still exist to get more value out of the developed AI solutions. Even after spending effort creating something like a chatbot, adoption can be difficult if the customer needs are not well understood. Solutions that fall into this capability are low-value / medium-effort solutions.
Process Automation
Some of the more commonly implemented AI technology solutions are completing specific tasks within a company that is part of an existing process or back-office system. The reason is that the particular functions are usually well understood and can be quickly reviewed and challenged by somebody familiar with completing the task. This also helps train the AI technology more efficiently. Some examples of automating processes are helping delivery drivers find faster routes, extracting specific information from legal documents, drafting communications, optimizing worker scheduling, or monitoring distracted driving. The effort involved in handling the automation of tasks ends up being pretty straightforward and, when put in production, can free up employees to focus on more critical work, which provides a lot of value. Solutions for the process automation capability usually end up being high-value / low-effort solutions, making them a higher priority than other capabilities for implementation.
Picking the Right Technologies
Once a company has identified the capability and solution that are being targeted, the next step is to identify the right technologies that should be utilized for implementation. Some technologies will help ensure that transparency of how decisions are made is available, which may be required if you’re working with highly regulated industry data. Other technologies focus more on cognitive decisions based on training and improving an AI model. These technologies offer less transparency and might provide a “black box” issue that needs to be navigated. Picking the right technologies can be the difference in going down the right path from the beginning vs. spending a large amount of effort on the wrong track using technologies that don’t support the needs of the solution.
Identifying and Prioritizing Opportunities
Which use cases offer the most short-term value and long-term value? How difficult is it to implement the proposed AI solution? Would the benefits be worth the effort? Answering these questions will help companies evaluate the implementation strategy and possibly even fail fast, realizing that addressing the problem is not all that critical to the overall strategy for the company. Once a company can identify the MVP for their target AI solution, the goal should be developing the AI solution quickly and launching a pilot in a controlled environment.
Challenges with Implementation
When attempting to implement an AI solution, many challenges will come up that can take time and resources that companies will need to navigate gracefully. Some of these challenges include building a data strategy. For example, what data will your business need? How much data will be required? How will data be collected, stored, and accessed? Who will ultimately be responsible for maintaining the data?
Building the right team can also be a challenge. Determining whether you need a data scientist or somebody with data analytics expertise is a decision that will depend on the identified technology and capability. The pool of experienced AI professionals is small as it's a newer focus, and finding the right talent to support a company’s AI implementation initiative can slow down a project before it even gets going.
If the solution requires cognitive training of an AI model, then understanding how your business can train an AI model in the first place is essential. Even after your business prepares an AI model, testing the AI model can also be a challenge, as companies will find varying results with AI models under different scenarios. One of the more difficult tasks when training AI models is what happens when you need to untrain an AI model due to undesirable results. These challenges occur at a critical stage in AI solution development.
Finally, after an AI solution is developed and tested, how does a company integrate the AI solution into company operations or monitor and evaluate the performance of the AI solution? How can a company continuously improve its AI solution? How companies navigate all of these challenges will determine how successful the implementation of AI technology is within an organization.
At Shift, we understand that there are many opportunities and challenges that come with implementing AI solutions. We love to help our clients determine the correct path. If you need support with AI, we’ve been there, and we got you. Start a conversation today.
This article was written by Travis Smith, Senior Software Developer